In 2008, the World Health Organization reported that major depressive disorder (MDD) was the third cause of global disability and predicted it to become the primary cause by 2030.1 By 2019, it became the second cause of global disability.1 Apart from its rising prevalence, MDD is known to be a very debilitating disease, causing patients to experience severe limitations in their physical, professional, and social capacities, leading to significant loss in quality of life and loss of productivity, thereby increasing the socioeconomic burden on the society.1,2
Identifying optimal treatment regimens for patients with MDD is important due to its significant influence on their overall outcomes and quality of life. Well-chosen MDD treatment plans, when identified and administered early in the course of the disease, are known to lead to the best outcomes with a lower risk of relapses.3 Each unsuccessful trial can compound the distress of the patient.3 Treatments need to be personalized, choosing from different modalities such as pharmacotherapy, psychotherapy, or their combination, based on the patient’s condition.4 Monitoring treatment efficacy and response is also essential so the treatment plans can be modified if the expected improvement is not observed.4
Electroconvulsive therapy (ECT) is a widely recognized and effective treatment for MDD, particularly for severe, treatment-resistant cases where a rapid response is necessary.5,6 ECT passes electric currents through the brain to induce a brief cerebral seizure. The consequent changes in brain chemistry help mitigate the depressive symptoms.7 ECT is associated with a 42% reduction in the risk of suicide within three months and a 28% reduction within 12 months for patients with MDD with psychotic features. The benefits of ECT extend beyond suicide risk reduction, as it has also been linked to reduced all-cause mortality at both three and 12 months.8 However, the response of patients to ECT is not homogeneous.7 Studies have identified predictors of ECT response, such as depression severity at baseline and age.9 For instance, post ECT remission in older patients has been associated with a 46% reduced risk of suicide, while remission in those aged 65 years and older has been associated with a 70% reduced risk of suicide.8 Work therapy (WT) and family therapy (FT) are also important components of MDD treatment. WT, or occupational therapy, can help individuals with MDD develop skills and strategies to manage their symptoms and improve daily functioning.5 FT can address interpersonal relationships and dynamics that may have contributed to the development or maintenance of MDD, fostering a supportive environment and improving communication for better treatment outcomes.5
While ECT, WT, and FT are valuable treatment options for MDD, it is important to recognize that not all methods may be equally effective for every patient.10 A person with specific characteristics might experience improvements following a particular treatment method, while others might remain unaffected or experience adverse events.11
Each individual with MDD may respond uniquely to interventions based on their personal characteristics, preferences, and needs.10 Therefore, finding the best method among ECT, WT, and FT or a combination of these, is crucial to maximize personalized treatment's benefits. For some, ECT might be the most effective option, especially in cases of severe, treatment-resistant depression where a rapid response is necessary. WT can be particularly beneficial for those struggling with daily functioning and facing occupational challenges due to their depression. FT can help manage interpersonal dynamics and foster a supportive environment for those whose relationships significantly impact their mental health. This can be achieved by healthcare providers working closely with patients to determine the most suitable and personalized treatment approach.12
In recent decades, personalized medicine has received much attention.10 By identifying optimal treatment rules for each patient based on their characteristics is the best way to obtain an effective treatment option that increases the probability of successful treatments and subsequently improves the patients' outcomes.2,13 Improving treatment outcomes such as reduced post treatment recovery time and number of hospitalizations can enhance physical health and quality of life of patients with psychiatric disorders.14,15
To our knowledge, no published study has attempted to determine individualized treatment regimens for patients with MDD among the options of WT, FT, ECT, or their combinations. Therefore, this study aimed to utilize the long-term health record data from north-western Iran to identify and characterize which patients are likely to derive the most benefit from different combinations of these treatments to improve health outcomes and reduce the number of hospitalizations.
Methods
The primary data16 was collected from a previous retrospective cohort study (non-randomized) which comprised 1005 patients who were diagnosed with MDD based on clinical interviews by psychiatrists according to the Diagnostic and Statistical Manual of Mental Disorders, Fifth Edition,17 and admitted to Farshchian Hospital in the city of Hamadan, Iran, during 2011–2018. For the current study, we selected a subset of 377 patients from among the 1005 MDD patients in the original study, using simple random sampling method. The demographic and clinical data pertaining to these 377 patients were extracted. The study received ethical approval from Hamadan University of Medical Sciences
(IR.UMSHA.REC.1400.936).
The selected participants were divided into the following four groups according to the treatment(s) they received: (1) WT, (2) WT + ECT, (3)WT + FT, and other psychotherapeutic treatments (PTs). The distribution of the treatment groups is shown in Tables 1 and 2.
Table 1: Treatments given to patients stratified by their demographic variables (N = 377).
History of recurrence
|
|
|
|
|
|
No
|
142 (53.8)
|
57 (21.6)
|
48 (18.2)
|
17 (6.4)
|
264
|
Yes
|
56 (49.6)
|
38 (33.6)
|
13 (11.5)
|
6 (5.3)
|
113
|
Gender
|
|
|
|
|
|
Male
|
86 (46.0)
|
52 (27.8)
|
42 (22.5)
|
7 (3.7)
|
187
|
Female
|
112 (59.0)
|
43 (22.6)
|
19 (10.0)
|
16 (8.4)
|
190
|
History of medication adherence
|
|
|
|
|
|
No
|
112 (53.3)
|
51 (24.3)
|
35 (16.7)
|
12 (5.7)
|
210
|
Yes
|
86 (51.5)
|
44 (26.3)
|
26 (15.6)
|
11 (6.6)
|
167
|
Living status
|
|
|
|
|
|
Parents
|
45 (47.4)
|
22 (23.2)
|
23 (24.2)
|
5 (5.3)
|
95
|
Others
|
153 (54.3)
|
73 (25.9)
|
38 (13.5)
|
18 (6.4)
|
282
|
Children
|
|
|
|
|
|
No
|
46 (46.5)
|
28 (28.3)
|
20 (20.2)
|
5 (5.1)
|
99
|
Yes
|
152 (54.7)
|
67 (24.1)
|
41 (14.7)
|
18 (6.5)
|
278
|
Marital status
|
|
|
|
|
|
Single
|
38 (48.1)
|
19 (24.1)
|
17 (21.5)
|
5 (6.3)
|
79
|
Married
|
160 (53.7)
|
76 (25.5)
|
44 (14.8)
|
18 (6.0)
|
298
|
Education level
|
|
|
|
|
|
No
|
132 (52.6)
|
59 (23.5)
|
45 (17.9)
|
15 (6.0)
|
251
|
Yes
|
66 (52.4)
|
36 (28.6)
|
16 (12.7)
|
8 (6.3)
|
126
|
Occupation
|
|
|
|
|
|
Unemployed
|
141 (54.0)
|
66 (25.3)
|
35 (13.4)
|
19 (7.3)
|
261
|
Employed
|
57 (49.1)
|
29 (25.0)
|
26 (22.4)
|
4 (3.4)
|
116
|
Location of residence
|
|
|
|
|
|
Urban
|
141 (52.2)
|
61 (22.6)
|
50 (18.5)
|
18 (6.7)
|
270
|
Rural
|
57 (53.3)
|
34 (31.8)
|
11 (10.3)
|
5 (4.7)
|
107
|
History of psychiatric illness
|
|
|
|
|
|
No
|
149 (52.1)
|
65 (22.7)
|
52 (18.2)
|
20 (7.0)
|
286
|
Yes
|
49 (53.8)
|
30 (33.0)
|
9 (9.9)
|
3 (3.3)
|
91
|
History of suicide
|
|
|
|
|
|
No
|
150 (54.3)
|
60 (21.7)
|
49 (17.8)
|
17 (6.2)
|
276
|
Yes
|
48 (47.5)
|
35 (34.7)
|
12 (11.9)
|
6 (5.9)
|
101
|
History of medical disorders
|
|
|
|
|
|
No
|
96 (47.8)
|
59 (29.4)
|
34 (16.9)
|
12 (6.0)
|
201
|
Yes
|
102 (58.0)
|
36 (20.5)
|
27 (15.3)
|
11 (6.5)
|
176
|
History of emotional problem
|
|
|
|
|
|
No
|
77 (52.7)
|
29 (19.9)
|
32 (21.9)
|
8 (5.5)
|
146
|
Yes
|
121 (52.4)
|
66 (28.6)
|
29 (12.6)
|
15 (6.5)
|
231
|
History of smoking
|
|
|
|
|
|
No
|
113 (55.9)
|
54 (26.7)
|
20 (9.9)
|
15 (7.4)
|
202
|
Yes
|
85 (48.6)
|
41 (23.4)
|
41 (23.4)
|
8 (4.6)
|
175
|
Type of discharge
|
|
|
|
|
|
Personal
|
45 (53.6)
|
22 (26.2)
|
12 (14.3)
|
5 (6.0)
|
84
|
WT + ECT: work therapy plus electroconvulsive therapy; WT + FT: work therapy plus family therapy; PT: other psychotherapy treatments.
Table 2: Additional variables and treatment characteristics of the patients (N = 377).
Age, years
|
40.2 ± 1.3
|
38.4 ± 1.3
|
39.8 ± 1.2
|
37.6 ± 1.2
|
39.6 ± 1.3
|
Recurrent number
|
0.7 ± 3.0
|
0.9 ± 1.7
|
0.3 ± 0.8
|
0.4 ± 0.9
|
0.7 ± 2.4
|
Duration of disorder, years
|
7.4 ± 8.3
|
7.2 ± 7.5
|
5.53± 5.9
|
6.2 ± 6.3
|
7.0 ± 7.6
|
Population, log
|
4.6 ± 1.1
|
4.4 ± 1.2
|
4.8 ±0.9
|
4.6 ± 1.0
|
4.6 ± 1.1
|
WT + ECT: work therapy plus electroconvulsive therapy; WT + FT: work therapy plus family therapy; PT: other psychotherapy treatments.
We included 19 baseline covariates. Of these, four were continuous variables: number of recurrences of MDD, age (years), duration of disorder (years), and the population size of the residential area (log population). The remaining 15 covariates were dichotomous and included: the history of recurrence, gender, medical disorder history, living status, number of children, marital status, education level, occupation, residence type, family history of psychiatric illnesses or mental disorders, suicide attempt history, medication adherence history, history of events associated with significant emotional problems (such as family members’ death/disease), history of smoking, and clearance type. All continuous covariates were standardized for analysis. For the dichotomous variables, we used dummy coding, assigning ‘1’ if present and ‘0’ if absent.
To identify the important variables and derive appropriate treatment modalities, we used the Penalized Regression Method which can perform these tasks simultaneously. We showed a loss-based estimation framework for estimating the optimal treatment regime. The steps of the Penalized Regression Method that we followed are detailed below:
Consider a data set of n, subjects from a clinical trial or observational study. The observed data include baseline patient characteristics, before treatment, denoted by vector X. The assigned treatment is represented by A = {0,1}. Let Y be the observed outcome of interest where we assume that higher values are preferred. Our goal is to establish an optimal treatment rule that maximizes the expected outcome Y, i.e., E(Y(g(X))). In this context, a treatment regime, g, is defined as a mapping from X into a treatment that would be assigned to an individual based on the observed covariates. For example, a patient with X = x is recommended treatment 1 if g(x) = 1, and so on.
We first define the concept of potential outcomes. Let Y(a) denote the potential outcome value under treatment a. We define Y(a) as the potential results if a patient were to receive treatment 1 or 0. Let G denote a class of all possible treatment regimens and g Є G an arbitrary treatment regime. We define the potential outcome under g, denoted (g) = Y (1).I (g (X) = 1) + Y (0).I (g (X) = 0).
Assuming full exchangeability, the average potential outcomes of patients who receive a treatment should be theoretically identical to those of patients who do not receive that treatment. This assumes that there are no unmeasured confounders influencing the outcome, except for the treatment itself. Under the assumption of consistency, the actual outcome (Y) will be independent of the treatment received by other patients.
The above two assumptions are likely to hold true in a randomized study, but their validity cannot be confirmed in a nonrandomized study. Rubin (1974)18 proposed that in both randomized and nonrandomized studies, the investigator should control the important variables that may causally affect Y. This control is to be achieved by matching, making adjustments, or combining both. Under these conditions, it can be shown that the expectation of the potential outcome, where Ex (.) represents the marginal distribution of X, can be expressed as
E (Y(a))
|
=
|
Ex [E (Y | X,A = a)]
|
In the class of all treatment regimens gopt ЄG it can be shown that
E (Y(g)) = E_X [E(Y | X, A = 1) g(X) + E(Y | A = 0,X) {1-g(X)}]
In our current regression model, we consider the linear form for the interaction effect, where
E(Y|X,A) = γX + A(β(X)), β = (β1,...,βp + 1)
The main objective is to estimate the interaction function βX but not the baseline function γX.19
To achieve the above objective, let Yi denote the observed outcome of interest; Xi the observed individual variables; AiЄ{0,1} the observed of assigned treatment to the individual, and π(ϰi) the propensity score. In a multiple regression model, parameters are typically estimated by applying loss functions, using the least squares method.20 Here, we apply the least squares method to minimize the loss function — where the minimizers are denoted as (γ͂,β͠) — as follows:

Given the observations (Yi, Xi, Ai, ei), new observations (Yinew, Xinew, Ai, ei) are constructed for every patient. Here, Yinew denotes the subtraction between Yi and values of baseline mean function of covariates, i.e., Yinew= Yi–γ͂ Xi, Xinew denotes the multiplication of Xi to {A_i-π(x_i)}, i.e.,
Ai denotes two available treatments A = {0,1} and ei denotes propensity scores for the treatment. Applying π(Xi) = p(Ai = 1 | Xi = xi),19,21 we obtain with a logistic regression model based on all the covariates, X.22
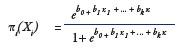
Finally, the standard quadratic form of loss function is formed as
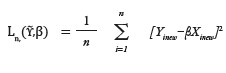
The minimizers are denoted as (β̂).
To estimate the optimal treatment rule, we estimated both the treatment effects and the interaction between the treatment covariates βΧ. The coefficients of these important variables (X) must be non-zero. The loss function makes it possible to achieve shrinkage penalties of coefficients, which aids in variable selection.23 This penalty term forces the model to select a small number of predictors, which should represent the most influential variables within the model. In this process, the model's coefficients are estimated by minimizing the loss function, which serves as an objective function.24
To select important prescriptive variables, we solved the equation
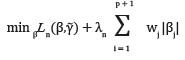
where λ is the tuning parameter and wj is the shrinkage penalty. We used adaptive Lasso penalty
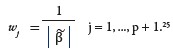
The adaptive Lasso penalty shrinks the coefficients towards zero, with the larger coefficients being shrunk more than the smaller coefficients.24
An optimal treatment rule depends on the treatment and prescriptive variables effects, βTX̃. It can be shown that the optimal treatment regime which patients receive with covariate X = x is
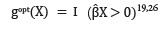
For example, in the model

the mean optimal rule is
Therefore, the variables with non-zero beta coefficients are named important variables.27
Results
The participants were 377 individuals diagnosed with MDD. Of them, 198 (52.5%) patients were treated with WT, 95 (25.2%) with WT + ECT, while 61 (16.2%) received WT + FT. The remaining 23 (6.1%) patients were treated with PT.
Tables 1 and 2 present the distribution of patient variables in the four treatment groups and negative number of hospitalizations. The response variable considered was the number of hospitalizations.28
We assumed that there would be heterogeneity in the outcome of treatments. The comparison of four treatment groups showed that the difference between the mean negative of number hospitalizations was significant (df = 3; F = 12.09; p < 0.010). There was also significant heterogeneity between the treatment groups (df1 = 3; df2 = 373; p ≤ 0.040). Therefore, it became necessary to identify the origins of heterogeneity and specify the unique characteristics of each individual instance of heterogeneity. For this purpose, we created a new dataset of matched groups, which in turn were based on propensity score predictions and the actual treatment administered to each patient.
Analysis I compared the effectiveness of WT (A = 1) to the combination of the other three treatments (A = 0) in 377 patients, of whom 198 (52.5%) received WT(A = 1) and 179 (47.5%) received the other three treatments (A = 0). To estimate the optimal treatment rule, we needed to show how variables affect the determination of the optimal treatment [Table 3]. For both constant and linear models in Analysis I, the estimated coefficients using adaptive Lasso penalty are given. One important covariate, history of emotional problems (h.emotional) which also included the death/disease of a family member or relative, was selected. Patients with h.emotional = 1 were more likely to receive the other three treatments (A = 0), which is reflected in the negative b*c value [Table 3]. This shows that the other three treatments for all patients with h.emotional = 1, is an optimal treatment rule. Based on the results, 61.3% of patients should receive the other three treatments (A = 0), whereas only 47.5% of patients were actually administered those.
Table 3: Estimated coefficients for interaction using the linear model.
Patient gender
|
0.00
|
–
|
–
|
–
|
0.00
|
–
|
–
|
–
|
Living status
|
0.00
|
–
|
–
|
–
|
0.00
|
–
|
–
|
–
|
Number of children
|
0.00
|
–
|
–
|
–
|
0.00
|
|
–
|
–
|
Marital status
|
0.00
|
–
|
–
|
–
|
0.00
|
–
|
–
|
–
|
Education level
|
0.00
|
–
|
–
|
–
|
4.02
|
|
1.00
|
4.02
|
Occupation
|
0.00
|
–
|
–
|
–
|
0.00
|
–
|
–
|
–
|
Type of residence
|
0.00
|
–
|
–
|
–
|
0.00
|
–
|
–
|
–
|
History of medication adherence
|
0.00
|
–
|
–
|
–
|
0.00
|
–
|
–
|
–
|
History of recurrence
|
0.00
|
–
|
–
|
–
|
0.00
|
–
|
–
|
–
|
History of psychiatric illness
|
0.00
|
–
|
–
|
–
|
0.00
|
–
|
–
|
–
|
History of suicide
|
0.00
|
–
|
–
|
–
|
0.00
|
–
|
–
|
–
|
History of medical disorders
|
0.00
|
–
|
–
|
–
|
0.00
|
–
|
–
|
–
|
History of emotional problem
|
-2.22
|
0.66
|
1.00
|
-2.22
|
3.68
|
0.94
|
1.00
|
3.68
|
History of smoking
|
0.00
|
–
|
–
|
–
|
0.00
|
–
|
–
|
–
|
Discharge type
|
0.00
|
–
|
–
|
–
|
0.00
|
–
|
–
|
–
|
Se: standard error; b: beta coefficient of covariates; c: variable value.
Optimal treatment rules were estimated by combining treatments to enhance the mean number of hospitalizations. A treatment rule in an interaction linear model depends on the coefficients of prescriptive variables. In analysis I, the negative coefficient implied a benefit from the other three treatments (treatment A = 0), if the linear combination yielded less than zero.
Analysis II compared WT + ECT (A = 1) to WT + FT (A = 0) in n = 156 patients, of whom 95 (60.9%) received WT + ECT (A = 1) and 61 (39.1%) received WT + FT (A = 0). Here, positive coefficient implied a benefit from the WT + ECT (treatment A = 1), provided the linear combination is > 0. We estimated the linear model’s coefficients using adaptive Lasso estimation. Two important covariates were selected: h.emotional and education level (edu).
Table 3 shows how these variables would influence the treatment decisions. Patients with h.emotional = 1 and edu = 1, h.emotional = 0 and edu = 1, or h.emotional = 1 and edu = 0 should receive WT+ECT (A = 1), as reflected in the positive b*c values [Table 3]. This suggested that for patients with h.emotional=1 and edu = 1, h.emotional = 0 and edu = 1, or h.emotional = 1 and edu = 0, treatment 1 (WT + ECT) was an optimal treatment rule. Thus, out of the 156 patients analyzed, 115 (73.7%) should have received treatment 1. However, only 95 (60.9%) patients were actually administered treatment 1.
For the constant model in Analysis II, the adaptive Lasso selected no important covariates, suggesting that WT + ECT (A =1) and WT + FT (A = 0) would be equally good for all patients in this study [Table 4].
Table 4: Estimated coefficients for interaction using the constant model in Analysis II.
Gender
|
0.00
|
–
|
–
|
–
|
0.00
|
–
|
–
|
–
|
Living status
|
0.00
|
–
|
–
|
–
|
0.00
|
–
|
–
|
–
|
Number of children
|
0.00
|
–
|
–
|
–
|
0.00
|
–
|
–
|
–
|
Marital status
|
0.00
|
–
|
–
|
–
|
0.00
|
–
|
–
|
–
|
Education level
|
0.00
|
–
|
–
|
–
|
0.00
|
–
|
–
|
–
|
Occupation
|
0.00
|
–
|
–
|
–
|
0.00
|
–
|
–
|
–
|
Type of residence
|
0.00
|
–
|
–
|
–
|
0.00
|
–
|
–
|
–
|
History of psychiatric illness
|
0.00
|
–
|
–
|
–
|
0.00
|
–
|
–
|
–
|
History of recurrence
|
0.00
|
–
|
–
|
–
|
0.00
|
–
|
–
|
–
|
History of medication adherence
|
0.00
|
–
|
–
|
–
|
0.00
|
–
|
–
|
–
|
History of suicide
|
0.00
|
–
|
–
|
–
|
0.00
|
–
|
–
|
–
|
History of medical disorders
|
0.00
|
–
|
–
|
–
|
0.00
|
–
|
–
|
–
|
History of emotional problem
|
-2.51
|
0.68
|
1.00
|
–2.51
|
0.00
|
–
|
–
|
–
|
History of smoking
|
0.00
|
–
|
–
|
–
|
0.00
|
–
|
–
|
–
|
Discharge type
|
0.00
|
–
|
–
|
–
|
0.00
|
–
|
–
|
–
|
Se: standard error ; b: beta coefficient of covariates c: variable value.
Optimal treatment rules were derived from the ‘Combining Treatments to Enhance’ study, to reduce the mean number of hospitalizations. The applicability of these treatment rules depended on the coefficients of predictor variables within a constant interaction model. In analysis I, a negative coefficient implied a benefit from the three treatments other than treatment A: WT + ECT, WT + FT, and PT (treatment A = 0) if the linear combination is less than zero. In analysis II, the absence of significant covariates implied that WT + ECT and WT + FT would be equally good for the 156 patients analyzed.
Discussion
This study enabled us to develop optimal treatment rules using a penalized regression-based estimation approach focusing on variable selection.29 Our results show that two important variables, emotional problems and education level, are the most significant for estimating the optimal treatment for MDD patients. Another study employing cluster analysis identified a subgroup of psychiatric patients who received ECT based on their characteristics.7 This was a non-homogeneous group but yielded three important variables, one of which was that older unemployed women with MDD had fewer ECT sessions.7 Our findings are consistent with that study’s results.
Further, the current study estimated three other treatment combinations—WT + ECT, WT + FT and PT—as optimal treatments for all patients with emotional problems. Administering WT alone was not deemed optimal. WT + ECT was identified as an optimal treatment for all patients, except for uneducated patients without a history of emotional problems. In similar studies conducted on patients with schizophrenia, it has been shown that employment may have positive effects on the cognitive function of patients.30 Another study found that neurocognitive enhancement therapy plus WT may modify performance on neuropsychological tests, but not WT alone, and our results are consistent with this.12
We concluded that combining ECT and WT for patients with the mentioned characteristics can reduce the number of hospitalizations and is an optimal treatment in MDD patients. A review of published works found ECT to be an efficient short-term treatment for reducing depression irrespective of other patient variables.31 The authors also found that various treatment approaches may be recommended for different subsets of MDD patients regardless of their characteristics and treatment efficacy.31 Both these findings align with those of the current study.
Sies et al,32 identified a treatment rule for optimal combinations of psychiatric medications for MDD patients based on their pretreatment characteristics, and its application improved their depression outcomes. In this cross sectional study dataset, the efficacy of two combination treatments was compared to that of a mono-treatment. Panic disorder problem and age were identified as two significant variables.32 With the weighted linear treatment regime, patients with panic disorder and patients < 28.5 years old should receive the combination treatment, whereas patients without panic disorder who are at least 28.5 years old should receive the mono-treatment.32,33 These findings also align with ours.
Most studies on MDD have focused on the efficacy of an individual therapy chosen from all available treatment options. However, our findings and those other studies cited above support combining different therapies based on treatment rules.
Research has also shown that although ECT is highly effective in protecting against MDD relapses, it needs to be augmented with depression-specific psychotherapeutic interventions such as cognitive-behavioral therapy and interpersonal therapy.34 However, these were not the focus of the current study as the number of patients who were treated using psychotherapy was too few for statistical analysis.
It may be relevant to make a mention an important tool, the Personalized Advantage Index (PAI), a multivariate approach to individualized treatment selection that developed by DeRubeis et al,33 in 2014. The PAI approach has been found to reasonably predict post-treatment scores for each treatment used by estimating the efficacy of an individualized treatment, comparing the relative advantage of an indicated treatment over a none-indicated treatment.33 In a recent study that used a regression model, the PAI of patients was calculated as an outcome. The optimal treatment was successfully predicted for 60% of patients (PAI score of ≥ 3).33 In another study, patients who received PAI-predicted optimal treatments developed fewer post-treatment symptoms than those who received treatments predicted as suboptimal.28
DeRubeis et al,33 and Friedl et al,28 also used PAI to identify important variables to determine the optimal treatment rules. They showed that important variables that could predict differential responses should be included in the regression model. More recently, van Bronswijk et al,10 used PAI to predict optimal individualized treatments to produce the better outcomes.10
Our study has limitations. Firstly, the sample size of 23 individuals in PT treatment group might be insufficient for multivariate models, limiting the generalizability of our findings. Secondly, some important variables such as the number of days of hospitalization were not considered in this study. Instead, we used the number of hospitalizations as an outcome.
Conclusion
This study estimated the optimal treatment rules for patients with major depressive disorder. We identified two significant individual variables: a patient’s history of emotional problems and educational level. For providing individualized treatment, important individual characteristics of a patient need to be identified and incorporated into the treatment regimen. This enables optimization of the treatment outcomes, including shortening the number of hospitalizations, and the likelihood of post-treatment complications.
Disclosure
The authors declared no conflicts of interest. The data is sourced from a Ph. D thesis in biostatistics by the first author, Narges Ghorbani, supported by a research grant from the Hamadan University of Medical Sciences, Hamadan, Iran
(Grant No. 1401010918).
Acknowledgments
We are grateful to the Vice-Chancellor of Education of the Hamadan University of Medical Sciences for approval and technical support.
references
- 1. Liu J, Liu Y, Ma W, Tong Y, Zheng J. Temporal and spatial trend analysis of all-cause depression burden based on global burden of disease (GBD) 2019 study. Sci Rep 2024 May;14(1):12346.
- 2. Karrouri R, Hammani Z, Benjelloun R, Otheman Y. Major depressive disorder: validated treatments and future challenges. World J Clin Cases 2021 Nov;9(31):9350-9367.
- 3. Seifert J, Maier HB, Führmann F, Bleich S, Stübner S, Sieberer M, et al. Pharmacological treatment of major depressive disorder according to severity in psychiatric inpatients: results from the AMSP pharmacovigilance program from 2001-2017. J Neural Transm (Vienna) 2022 Jul;129(7):925-944.
- 4. Gautam S, Jain A, Gautam M, Vahia VN, Grover S. Clinical practice guidelines for the management of depression. Indian J Psychiatry 2017 Jan;59(Suppl 1):S34-S50.
- 5. Xin Y, Bai T, Zhang T, Chen Y, Wang K, Yu S, et al. Electroconvulsive therapy modulates critical brain dynamics in major depressive disorder patients. Brain Stimul 2022;15(1):214-225.
- 6. Abbott CC, Lemke NT, Gopal S, Thoma RJ, Bustillo J, Calhoun VD, et al. Electroconvulsive therapy response in major depressive disorder: a pilot functional network connectivity resting state FMRI investigation. Front Psychiatry 2013 Mar;4:10.
- 7. Al Saadi A, Chan MF, Al-Kaabi S, Al Shukaili M, Al-Mamari F, Al Abdali M, et al. Demographic and clinical profile of patients receiving electroconvulsive therapy at a tertiary care hospital in Oman: a cluster analysis. Oman Med J 2022 Jul;37(4):e401.
- 8. Gaynes BN. Benefits of electroconvulsive therapy for patients with major depressive disorder. JAMA Network Open. 2021;4(7):e2116674.
- 9. Su L, Zhang Y, Jia Y, Sun J, Mellor D, Yuan T-F, et al. Predictors of electroconvulsive therapy outcome in major depressive disorder. Int J Neuropsychopharmacol 2023 Jan;26(1):53-60.
- 10. van Bronswijk SC, DeRubeis RJ, Lemmens LH, Peeters FP, Keefe JR, Cohen ZD, et al. Precision medicine for long-term depression outcomes using the personalized advantage index approach: cognitive therapy or interpersonal psychotherapy? Psychol Med 2021 Jan;51(2):279-289.
- 11. Qian M, Murphy SA. Performance guarantees for individualized treatment rules. Ann Stat 2011 Apr;39(2):1180-1210.
- 12. Bell M, Bryson G, Greig T, Corcoran C, Wexler BE. Neurocognitive enhancement therapy with work therapy: effects on neuropsychological test performance. Arch Gen Psychiatry 2001 Aug;58(8):763-768.
- 13. Stefanicka-Wojtas D, Kurpas D. Personalised medicine-implementation to the healthcare system in Europe (focus group discussions). J Pers Med 2023 Feb;13(3):380.
- 14. Mamani M, Majzoobi MM, Ghahfarokhi SM, Esna-Ashari F, Keramat F. Assessment of health-related quality of life among patients with tuberculosis in Hamadan, Western Iran. Oman Med J 2014 Mar;29(2):102-105.
- 15. Phang K, Abdul Latif AA, Lee KW, Ching SM, Ooi PB. Effects of psychotherapy on quality of life in end-stage renal disease patients: a systematic review with meta-analysis. Oman Med J 2022 May;37(3):e383.
- 16. Parami S, Tapak L, Poorolajal J, Moghimbeigi A, Ghaleiha A. Identifying factors associated with the hospital readmission rate among patients with major depressive disorder. BMC Psychiatry 2021 Nov;21(1):542.
- 17. Edition F. Diagnostic and statistical manual of mental disorders. Am Psychiatric Assoc. 2013;21(21):591-643.
- 18. Rubin DB. Estimating causal effects of treatments in randomized and nonrandomized studies. J Educ Psychol 1974;66(5):688.
- 19. Lu W, Zhang HH, Zeng D. Variable selection for optimal treatment decision. Stat Methods Med Res 2013 Oct;22(5):493-504.
- 20. Taboga M. Lectures on probability theory and mathematical statistics; 2017.
- 21. Gunter L, Zhu J, Murphy SA. Variable selection for qualitative interactions. Stat Methodol 2011 Jan;1(8):42-55.
- 22. Zhang B, Tsiatis AA, Laber EB, Davidian M. A robust method for estimating optimal treatment regimes. Biometrics 2012 Dec;68(4):1010-1018.
- 23. Geng Y, Zhang HH, Lu W. On optimal treatment regimes selection for mean survival time. Stat Med 2015 Mar;34(7):1169-1184.
- 24. Zou H. The adaptive lasso and its oracle properties. J Am Stat Assoc 2006;101(476):1418-1429.
- 25. Gunes F. Penalized regression methods for linear models in SAS/STAT®. Proceedings of the SAS Global Forum 2015 Conference Cary, NC: SAS Institute Inc http://support sas com/rnd/app/stat/papers/2015/PenalizedRegression_LinearModels pdf; 2015.
- 26. Fu H, Zhou J, Faries DE. Estimating optimal treatment regimes via subgroup identification in randomized control trials and observational studies. Stat Med 2016 Aug;35(19):3285-3302.
- 27. Wang L, Zhou Y, Song R, Sherwood B. Quantile-optimal treatment regimes. J Am Stat Assoc 2018;113(523):1243-1254.
- 28. Friedl N, Krieger T, Chevreul K, Hazo JB, Holtzmann J, Hoogendoorn M, et al. Using the personalized advantage index for individual treatment allocation to blended treatment or treatment as usual for depression in secondary care. J Clin Med 2020 Feb;9(2):490.
- 29. Zhang B, Zhang M. Variable selection for estimating the optimal treatment regimes in the presence of a large number of covariates. Ann Appl Stat 2018;12(4):2335-2358.
- 30. Hasbollah NN, Abu Bakar AK, Shuib N, Jamil AT, Razali S, Md Som S. Maintaining functional working memory through job activities: a study among patients with schizophrenia enrolled in a supported employment program. Journal of Vocational Rehabilitation 2022 Jan 1;57(2):141-150.
- 31. Group UE; UK ECT Review Group. Efficacy and safety of electroconvulsive therapy in depressive disorders: a systematic review and meta-analysis. Lancet 2003 Mar;361(9360):799-808.
- 32. Sies A, Van Mechelen I. Estimating the quality of optimal treatment regimes. Stat Med 2019 Nov;38(25):4925-4938.
- 33. DeRubeis RJ, Cohen ZD, Forand NR, Fournier JC, Gelfand LA, Lorenzo-Luaces L. The personalized advantage index: translating research on prediction into individualized treatment recommendations. A demonstration. PLoS One 2014 Jan;9(1):e83875.
- 34. McClintock SM, Brandon AR, Husain MM, Jarrett RB. A systematic review of the combined use of electroconvulsive therapy and psychotherapy for depression. J ECT 2011 Sep;27(3):236-243.